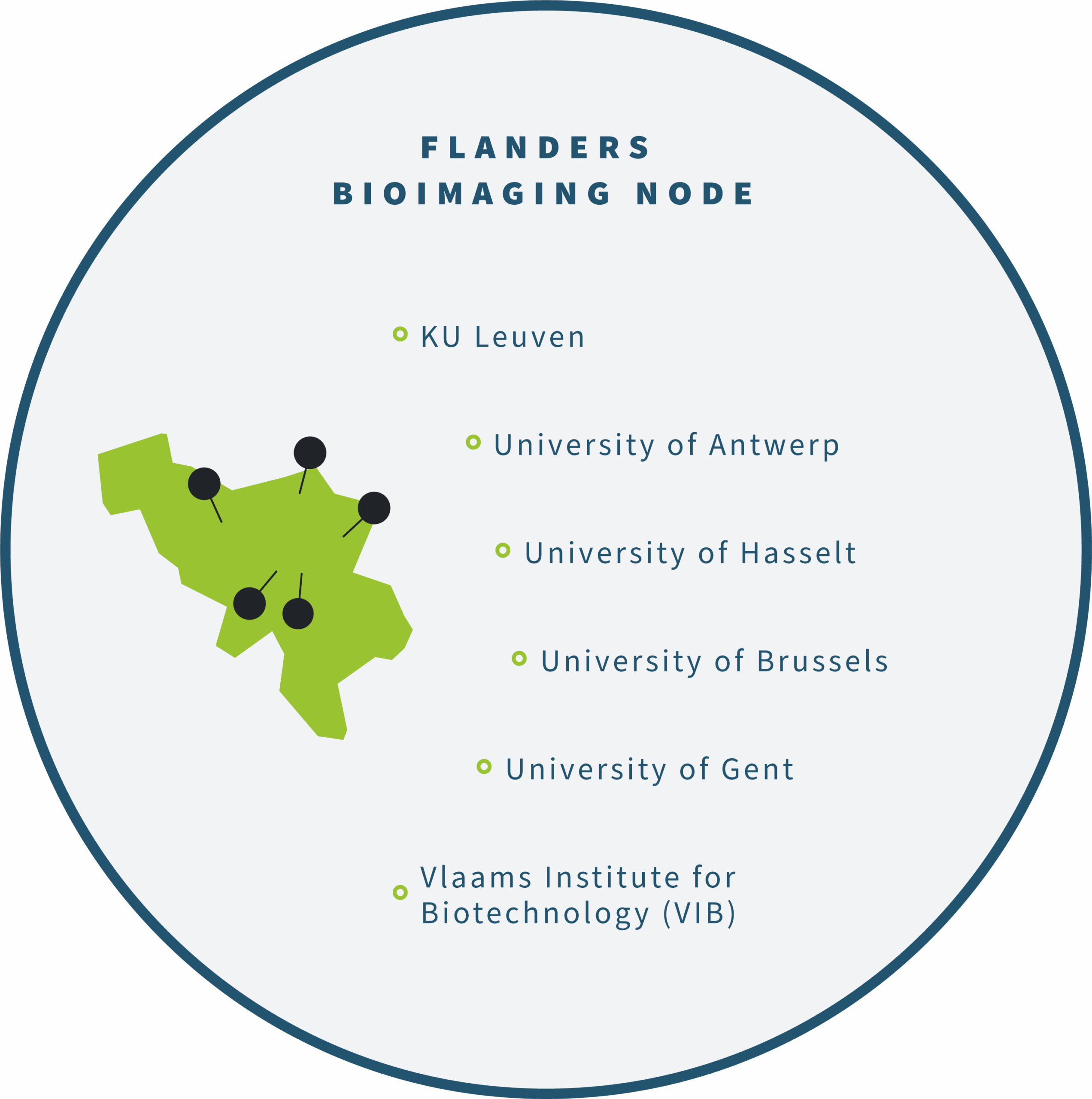
Image data services interview at the BioImage Informatics Facility (BIIF), part of Swedish NMI
Imaging scientists are producing datasets of increasing volume, complexity, and information content. To realize the full potential of imaging data, Euro-BioImaging Nodes offer a range of Image Data Services, including data handling, developing bespoke image analysis workflows, quantitative analysis, and access to analysis software. Access to these services is currently available at selected Nodes as part of our Proof-of-Concept study. To learn more about Swedish NMI Node’s expertise and services, we spoke to Anna Klemm of the BioImage Informatics Facility (BIIF).
What type of image data services do you and your team offer?
Besides organizing seminars, training and educational events, which we provide free of cost to the community, BIIF provides support to researchers in the development of image analysis workflows. Our support can be categorized into three main types of projects:
(a) Simple projects, which can be handled by consultancy meetings to discuss software solutions and doubts related to image acquisition and analysis;
(b) Typical projects, which involve education and development. Scientific papers resulting from such projects can lead to co-authorship for BIIF members; and,
(c) Long-term projects, which involve funded support through user fees, allocation of staff research time with the possibility of joint external funding, and also industry collaborations.
PI located in Sweden receive 20 hours free support, which can become long-term support projects. A general overview of the research projects that BIIF is supporting is available on our project list (https://biifsweden.github.io/).
What is a typical project like?
Here’s an example of a long-term project we’ve been working on with a user from Karolinska Institutet since 2020. Let’s call it “Neighborhood analysis in glioma tissue.” In this project we developed an automated approach to classify multiplexed image data of cells into different cell types and analyze spatial relationships between cell types in brain tumor tissue slices. Users detected cells automatically in QuPath and annotated a training set regarding cell types. Using Neural Networks in Python all cells in the data set were classified and the results exported to QuPath for further visual control. This project started as a pure user project and later involved a PhD- student of the Wählby group, UU. It lead to a publication: L. Solorzano et al., “Machine learning for cell classification and neighborhood analysis in glioma tissue,” Cytometry Part A, doi: 10.1002/cyto.a.24467, in which we are co-authors.
Here's another example of a long-term project which we will call “Quantifying Cancer-induced vascular changes.” In this case, the users were from Uppsala University. As you know, lymph node tumor metastasis is one of the most important prognostic factors in human cancer and correlate with an increased risk for further metastatic spread in several types of cancer, including breast cancer and pancreatic cancer. In this project, the user had identified cancer-induced vascular changes that can provide value as biomarkers. BIIF developed a Deep Learning based software for detecting these cancer-induced vascular changes. Using a Mask-RCNN network model - a deep neural network aimed to solve instance segmentation problems in machine learning - trained on more than 16000 manually annotated vessels from 80 patients, the software can detect and classify vessels depending on their dilation. The software also detects fat, metastases, and regions positive for a specific cell marker in the lymph nodes. A graphical user interface allows the user to apply the model to new images, and export all annotations as Regions of Interest for ImageJ and/or QuPath, two open-source tools widely used in life science research. See the publication: Bekkhus et al.,“Automated detection of vascular remodeling in tumor-draining lymph nodes by the deep-learning tool HEV-finder,” in The Journal of Pathology, doi: https://doi.org/10.1002/path.5981
How can we get started?
Typically a potential user contacts us by e-mail or via the NBIS support form (https://nbis.se/support/supportform/index.php). Since BIIF is a Swedish national facility, we rely on e-access. We will set up initial meetings to learn more about the project and we will work together mainly via zoom. If the project is deemed feasible, all data will be transferred via shared folders. Researchers located in Sweden can apply for one of the generous grants from Knut and Alice Wallenberg Foundation (KAW), which finances a senior bioinformatician that will work on your project 500 hours for free. Applications are evaluated every four months by an external committee.
More news from Euro-BioImaging
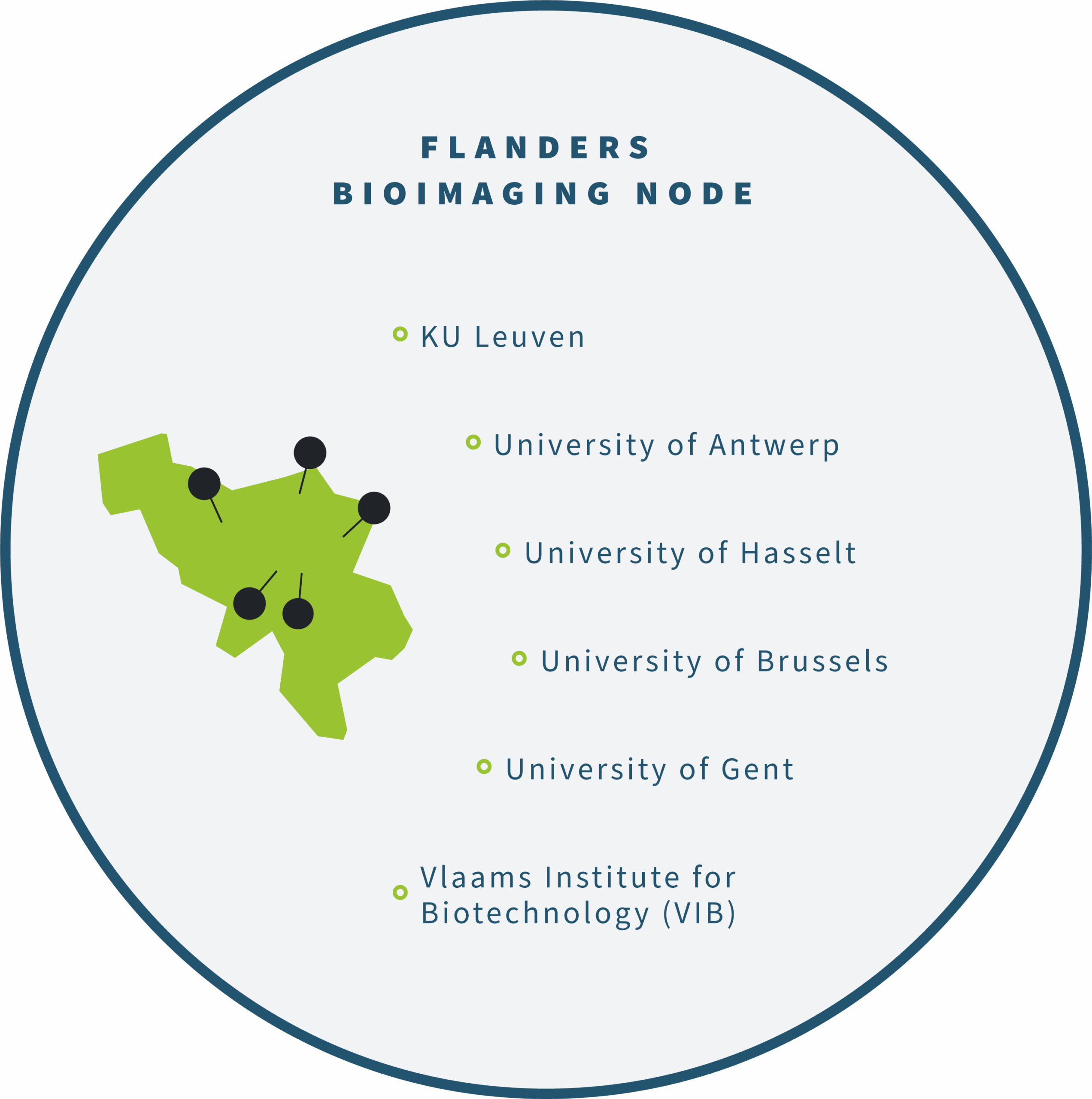
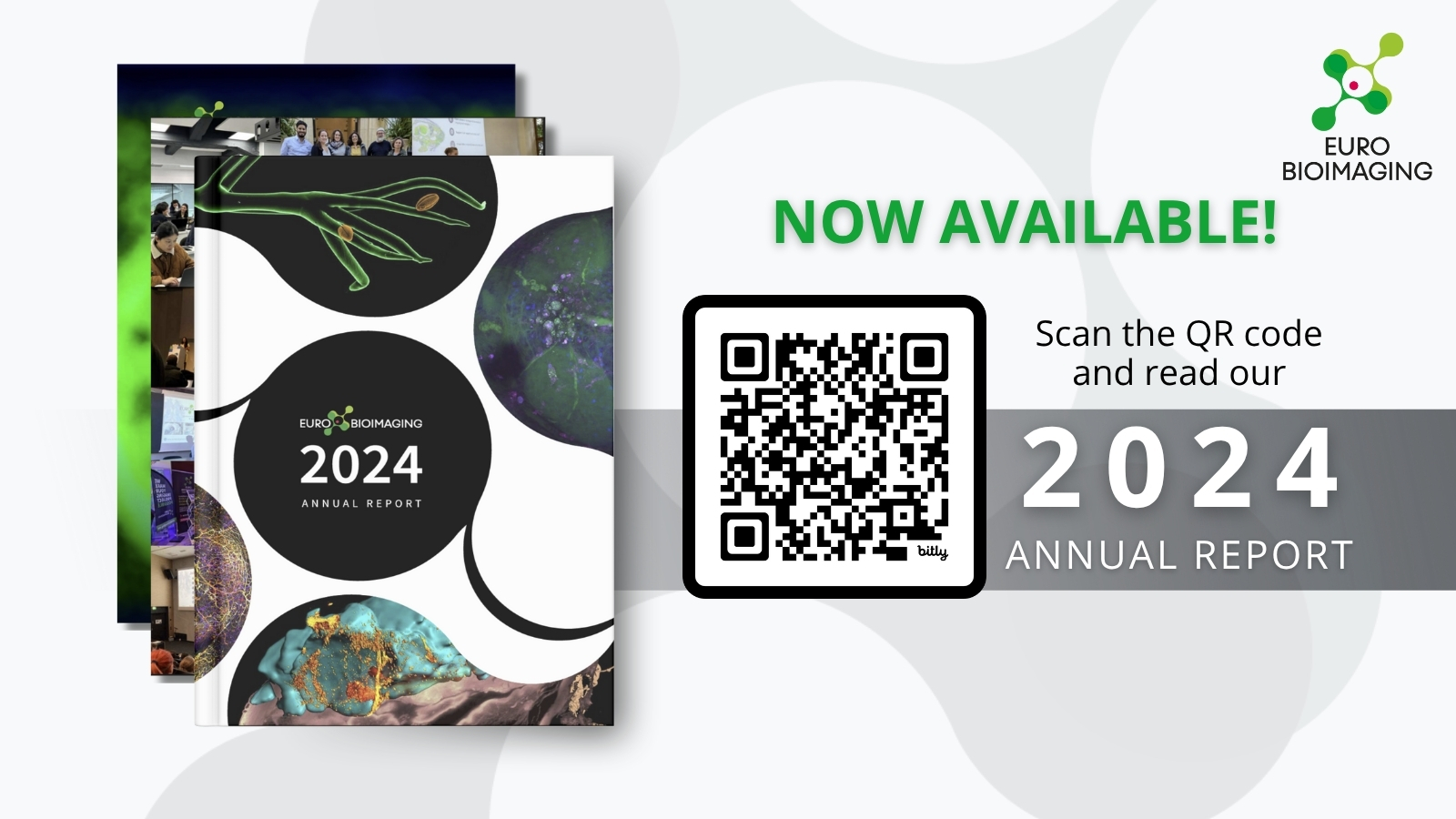
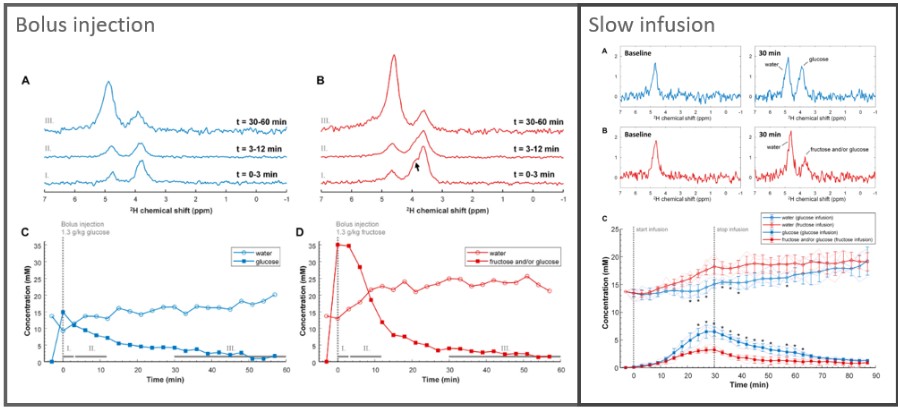